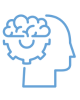
Artificial intelligence can refer to a variety of technology-assisted applications. At its essence, it refers to systems or machines that mimic human intelligence to perform tasks and, in some cases, that can iteratively improve themselves based on the information they collect. In healthcare, the applications of AI that we see most often are:
- Bots, or digital workers, handling time-consuming, repetitive, mind-numbing tasks prone to transaction errors.
- Intelligent assistants using AI to parse clinical information from free text notes to identify diagnoses, symptoms, and treatments not represented in discrete fields.
- Pattern recognition algorithms scanning text and digital images such as notes, x-rays, CT scans, and MRI images to identify lesions, nascent tumors and other abnormalities difficult to identify with the naked eye.
However, AI comes with numerous challenges that, if not identified and corrected, can generate incorrect findings and, at worst, threaten patient health and safety.
The data problem
Training AI systems requires large amounts of data from sources such as electronic health records, pharmacy records, medical images, insurance claims records, remote monitoring technology, and consumer health devices. Unfortunately, this data is typically fragmented across many different systems. Aside from the variety of sources just mentioned, patients typically see different providers, switch insurance companies, and change home devices, leading to their data being split into multiple systems using many different formats. This fragmentation increases the risk of error, decreases the comprehensiveness of datasets, and increases the expense of gathering data—which also limits the types of entities that can develop effective healthcare AI.Additionally, health data is often coded in ICD-10 or CPT codes; these are billing rather than clinical codes. When researchers use ICD-10 or CPT codes, they are applying data designed not for clinical research but to comply with the reimbursement requirements of health plans. A patient presenting to the emergency department with a bite on their hand is just as likely to be coded with a bruise or a contusion because the reimbursement is unaffected.
Pertinent data isn't being collected for several reasons. Clinicians frequently work in settings that are busy and distracting. Patients may have difficulty describing their symptoms, medications, and other clinical information accurately and completely. Patients have multiple complaints and symptoms which may or may not be interrelated; they may not get the chance to discuss all of them with their clinician before time runs out in an appointment.
Further, electronic medical records are complex and not designed to assist clinicians in dealing with the information patients present. The need to look at a computer screen may distract clinicians or inhibit patients from talking freely, meaning useful data could be missed.
The privacy (and resulting trust) problem
AI has the capability of predicting private information about patients even if the patient has never shared or consented. As an example, Parkinson’s disease could be diagnosed (possibly incorrectly) by an AI system because a patient’s hand trembles as they use the mouse. Other AI applications, such as those being tested for the diagnoses of mental disorders, present the same potential for invading a patient’s privacy and destroying their trust in the health system.Financial transaction data might show that a patient eats lunch at a cancer hospital twice a week. An AI system could use this to decide they probably have cancer (or signs of cancer) rather than they work next door, or volunteer, or whatever the real story is. Similarly, AI could analyze a patient's transactions at a pharmacy and decide they have diabetes based on a medication they take for something else, most often used by diabetics. Both of these cases could lead to enrollment in inappropriate case management programs, outreach to the patient erroneously assuming they have a medical condition, and other actions that erode trust in the system.
The bias and inequality problem
Bias is introduced at every stage of AI development and use. AI systems learn from their training data and absorb biases found in this data. Studies show that AI developed with data from a particular setting or constituency (such as academic medical centers or Medicare patients) is most effective for that population and not always effective for others. Even if AI systems learn from accurate, representative data, there can still be problems if that information reflects underlying biases and inequalities in the health system. For example, African American patients receive, on average, less treatment for pain than white patients. An AI system learning from health system records might learn to suggest lower doses of painkillers to African American patients even though that decision reflects systemic bias, not a biological reality. Resource allocation AI systems could also increase inequality by assigning fewer resources to patients considered less desirable or less profitable by health systems for various problematic reasons.
Algorithms for machine learning are often chosen by trying several options and choosing the best fit. Humans are making that choice, bringing their own biases to it. Further, if they don't understand the potential biases from each possible approach they can't be considered in the selection process. People also make decisions about thresholds for matches and other aspects of an application that affect its results; these, too, can introduce new or promulgate existing biases.
In addition, bias and inequity can result from the way AI is applied to patients. Many people don't understand that AI is inherently probabilistic, how their results come to be, and how they are best interpreted. Someone, usually without clinical expertise, is deciding what's good enough to flag as a match. They don't always weigh risks of including extra matches or omitting too many potential matches. When physicians apply these results to their patients, they need to consider this and act accordingly, not just assume that the AI accurately identified whether or not a patient has X or needs Y.
What we're doing about it
MHDC is working with payers and providers to address several of these AI problems. Our Data Governance Collaborative is increasing the adoption of common clinical vocabularies and application programming interfaces that enable the sharing of information among organizations. These efforts produce more coherent and structured clinical information and reduce the risks associated with the current fragmentation of clinical data across numerous systems, within and outside the healthcare enterprise. It also explores how AI works, some of the ways bias can be introduced, and how the industry as a whole can try to minimize them.
We are also working on automating the burdensome process of prior authorization. We are working with a leading payer and specialty provider to identify and document when prior authorization is required, reducing the volume of prior authorization requests the provider submits to the payer. Part of this work is aimed at replacing bots that try (and fail) to make complex operations routine (such as accessing multiple payer portals for prior authorization information) with simpler and more direct machine-to-machine exchanges that are more robust, reliable, and scalable.
We are also actively participating in industry discussion around AI including recent sessions where ONC solicited ideas around how to improve AI use in healthcare. We provided suggestions in several areas including data collection, reporting, education, and technical and user support lines. Don't be surprised if new certification requirements and other HHS regulations or programs show up around AI.
MHDC believes that AI has the potential to dramatically improve clinical care, reduce administrative complexity, and improve the patient and member experience. We are mindful of the risks that ill-considered and imprudent use of AI can present and, by collaborating with numerous constituents, are confident we can navigate this complex process successfully.